Our most recent research article, titled “Advancements in hand-drawn chemical structure recognition through an enhanced DECIMER architecture,” has been published in the Journal of Cheminformatics. In this work, we present an enhanced Deep lEarning for Chemical ImagE Recognition (DECIMER) architecture that leverages a combination of Convolutional Neural Networks (CNNs) and Transformers to improve the recognition of hand-drawn chemical structures. The model incorporates an EfficientNetV2 CNN encoder that extracts features from hand-drawn images, followed by a Transformer decoder that converts these extracted features into Simplified Molecular Input Line Entry System (SMILES) strings. Our models were trained using synthetic hand-drawn images generated by RanDepict, a tool for depicting chemical structures with different style elements. A benchmark was performed using a real-world dataset of hand-drawn chemical structures to evaluate the model’s performance. The results indicate that our improved DECIMER architecture exhibits significantly enhanced recognition accuracy compared to other approaches. All the resources can be found at the following links:
- Code availability: https://github.com/Kohulan/DECIMER-Image_Transformer.
- Model availability: https://doi.org/10.5281/zenodo.10781330.
- PyPi Package: https://pypi.org/project/decimer/.
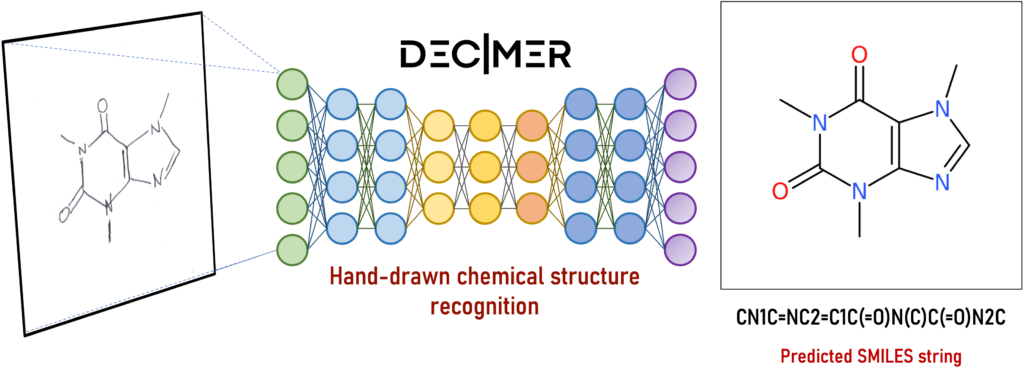
Rajan, K., Brinkhaus, H.O., Zielesny, A. et al. Advancements in hand-drawn chemical structure recognition through an enhanced DECIMER architecture. J Cheminform 16, 78 (2024). https://doi.org/10.1186/s13321-024-00872-7